Enchant v2: Pushing the Boundaries of Predictive Drug Discovery
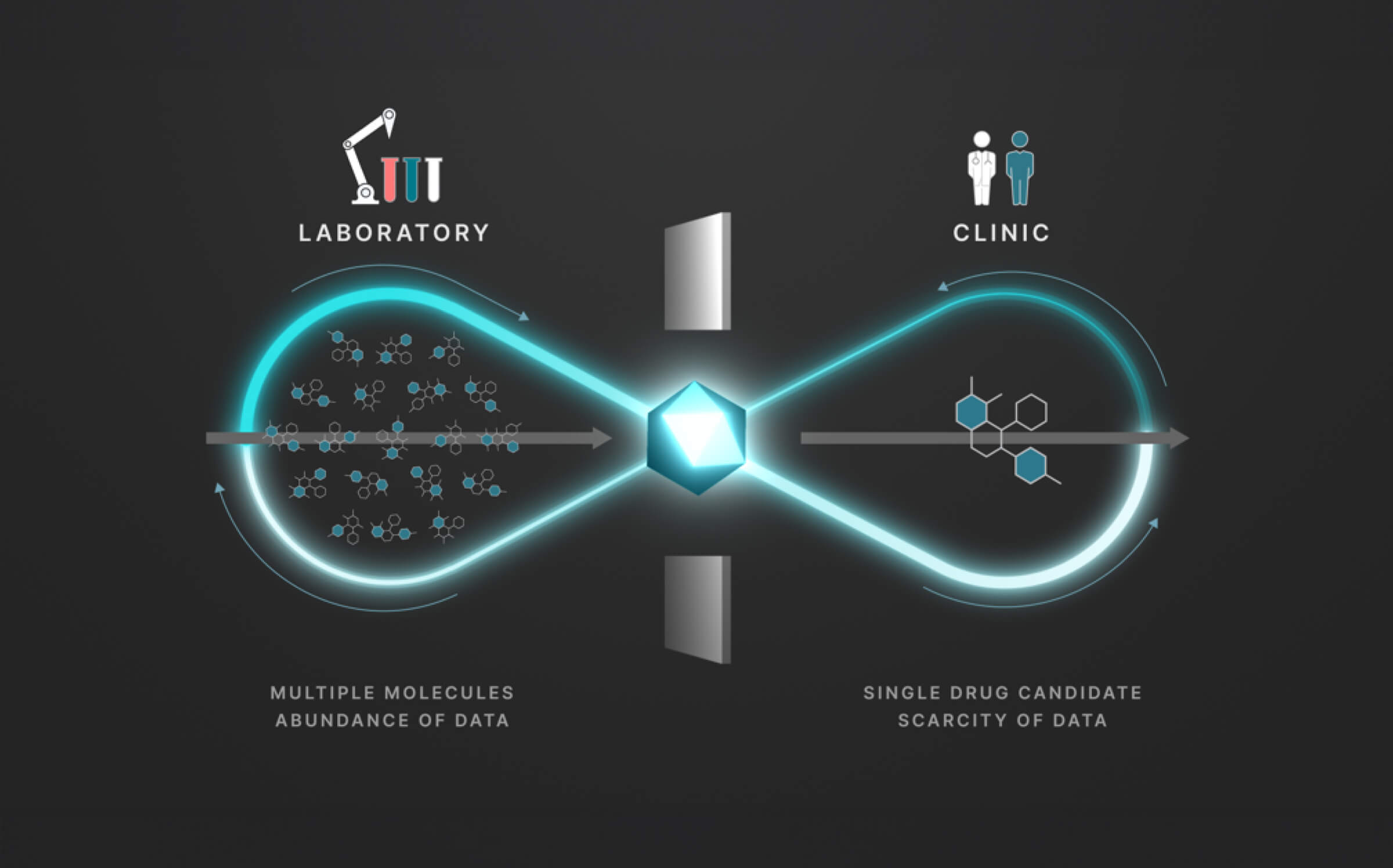
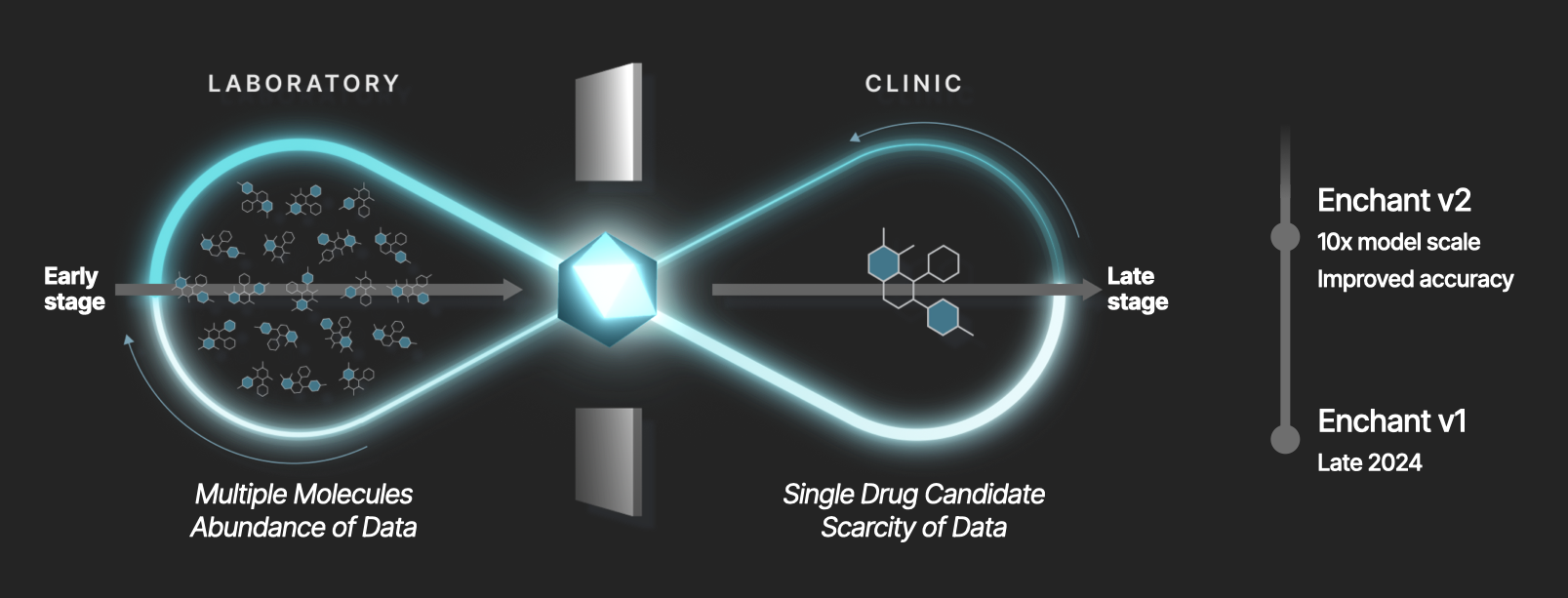
Last year, we introduced Enchant, a multimodal transformer model that redefined drug discovery prediction in low-data regimes. Enchant is trained on many data modalities from dozens of external and internal sources, across chemical, biological, preclinical, clinical, and other contexts. The ability to make high-confidence predictions in low-data regimes is of particular importance to drug discovery, where the capacity to generate data at scale is limited to the earliest stages of the discovery process.
Today, we are excited to announce the next generation of this technology: Enchant v2 is a major advance in model scale, predictive performance, and real-world impact. Powered by a greater-than-tenfold increase in model scale, and a series of architectural and training innovations, Enchant v2 sets new benchmarks across diverse molecular property prediction tasks. Enchant is pushing the boundaries of drug discovery, with high-confidence predictions in low-data regimes enabling:
- Multi-parameter optimization around important drug properties
- Prioritization and resource allocation to programs based on predicted endpoints
- Design of clinical trials for the rapid translation of potential therapeutics
Superior Predictive Accuracy Across Key Properties
To rigorously evaluate Enchant v2, we benchmarked its performance on the Biogen ADME benchmark, alongside Enchant v1 and TxGemma, the recently announced model from Google DeepMind based on Gemma 2.1
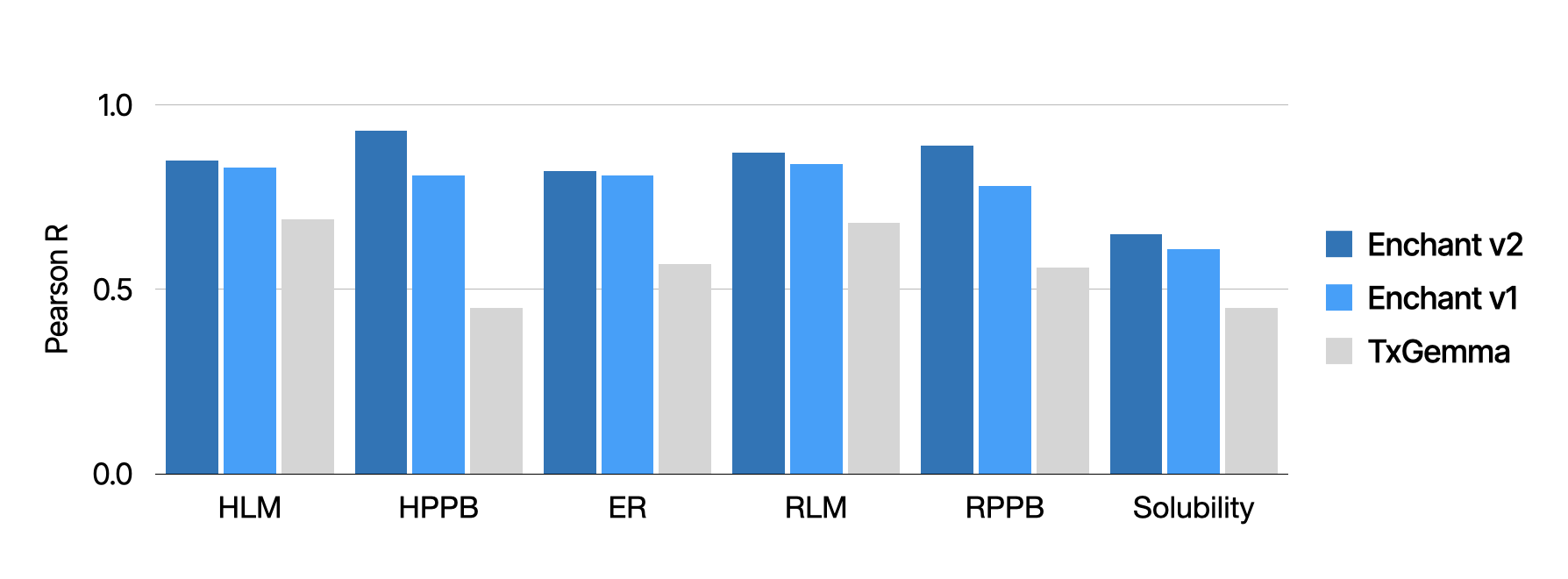
Across all endpoints in the benchmark Enchant v2 consistently outperforms both Enchant v1 and TxGemma. Notably, Enchant v2 achieved improvements even in notoriously difficult endpoints like plasma protein binding and solubility, further extending its utility for drug candidates entering preclinical development. Moreover, Enchant is able to make high-confidence predictions across many biological, physiochemical, pharmacokinetic, metabolic, safety, and other properties, helping advance Iambic’s internal programs and partnership activities.
Enchant is the new state-of-the-art model for Kinase200
Enchant v2 is a powerful model for learning from small and noisy data sets to make informative predictions across a huge range of biochemical and cellular activities, and for a wide range of target classes. Here we demonstrate that Enchant v2 is the new state-of-the-art model for predicting kinase activities via the Kinase200 benchmark, outperforming competitor models by a large margin. On the Kinase200 benchmark, Enchant v2 delivered a median Pearson R of 0.69, significantly exceeding Enchant v1 (0.61) and TxGemma (0.55).
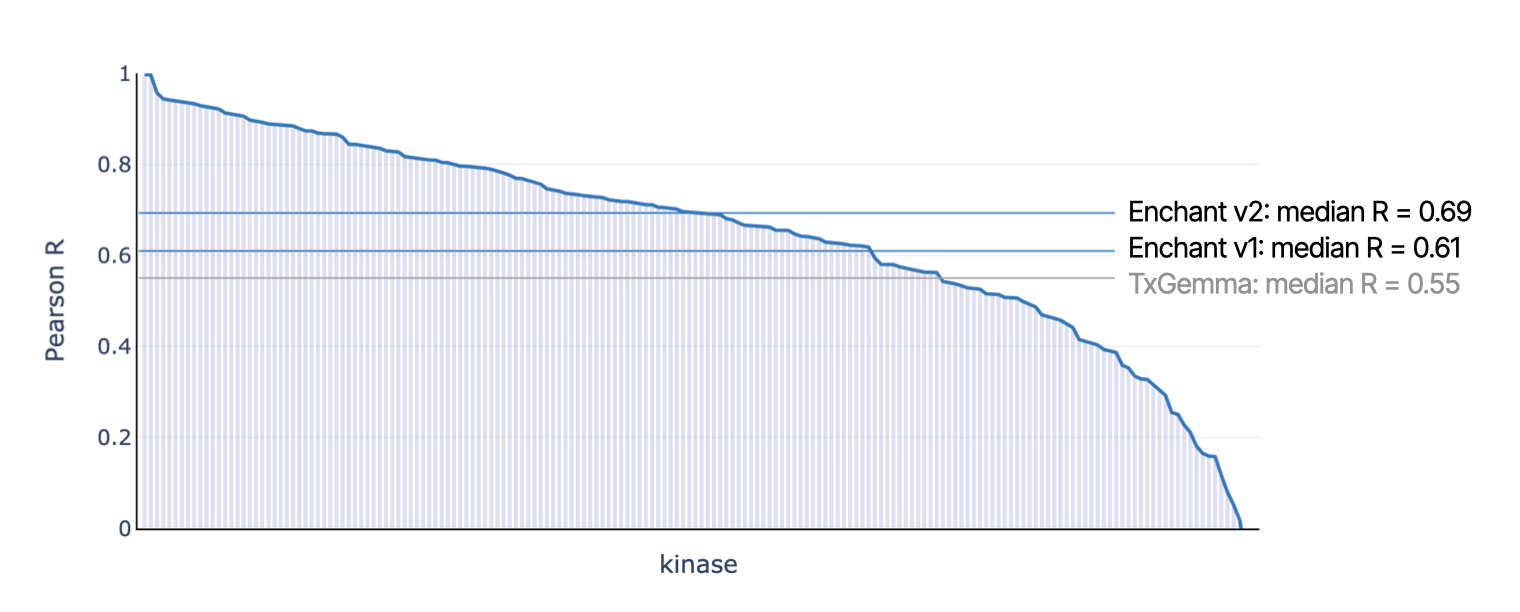
Broad Impact Across Real-World Discovery Endpoints
Beyond retrospective benchmarks, Enchant v2 has been fine-tuned on dozens of properties associated with discovery programs at Iambic. Here we compare Enchant v2, Enchant v1 and TxGemma across diverse examples, such as transport properties, biochemical inhibition, cellular pharmacodynamics, and solubility. For each case, the performance ordering Enchant v2 > Enchant v1 > TxGemma is maintained.
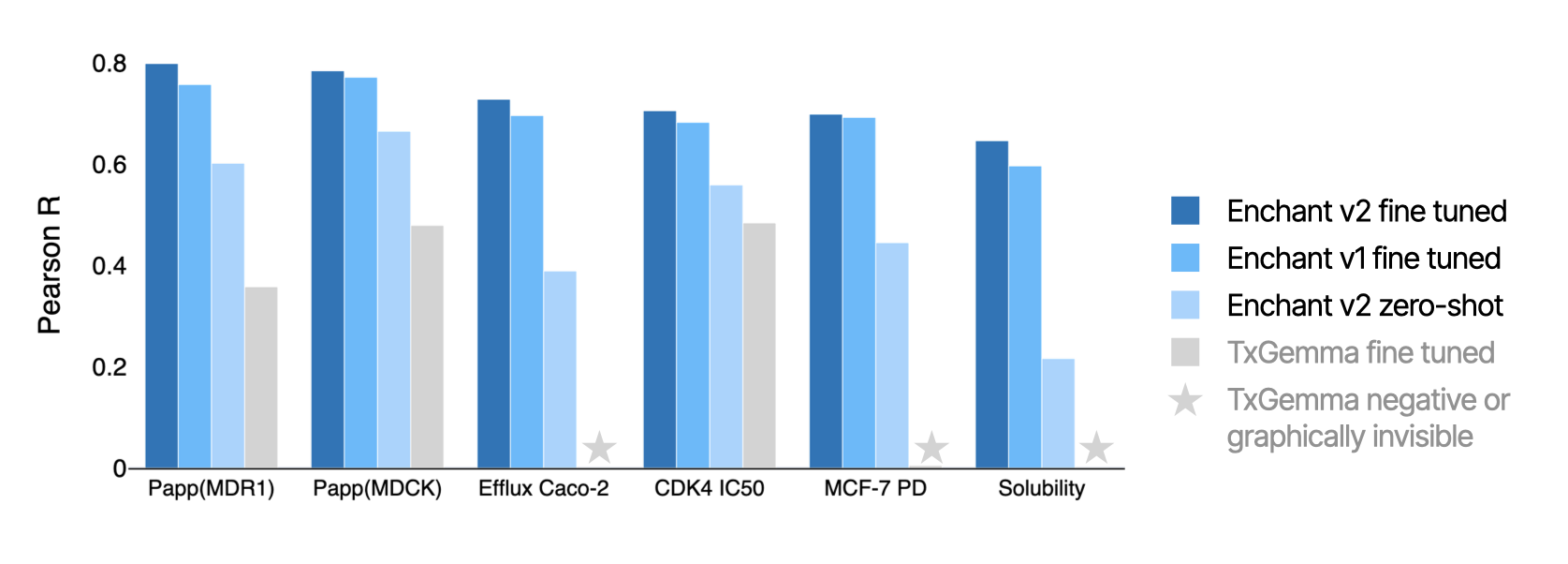
Remarkably, Enchant v2 zero-shot predictions — predictions made without any fine-tuning of the Enchant v2 foundation model — also proved surprisingly reliable, greatly improving over the zero-shot performance of Enchant v1. It is noteworthy that the zero-shot Enchant v2 performance surpasses fine-tuned TxGemma by a considerable margin. For example: the Enchant v2 foundation model saw MCF-7 PD data for just 12 distinct molecules, and yet zero-shot inference of this property on held-out molecules achieves a Pearson R = 0.45. This zero-shot capacity enables immediate predictive signal on newly added assays in emerging programs, and underlines the performance of Enchant in the low-data regime.
How Enchant is changing drug discovery
A critical goal of the Enchant project is to break the data wall between preclinical discovery (where data can be manufactured at scale) and clinical development (where it cannot). In our earlier post, we demonstrated how Enchant predictions of human pharmacokinetic (PK) half-life can be improved by training on successive classes of preclinical data spanning in vitro data, mouse PK, and higher-species preclinical PK. Here we illustrate the power of Enchant to learn directly from a random sampling of preclinical data, highlighting a key attribute of this technology: Enchant gets better at predicting clinical endpoints by being trained on more preclinical data.

In many scenarios in real-world drug discovery, data density (i.e., what is known about a particular biological target or chemical mechanism of action) can be particularly low. In one Iambic program, we noticed a significant disconnect between in vitro and in vivo clearance. Enchant, fine-tuned on a small set of in vivo pharmacokinetics data set together with more abundant in vitro data, is a better predictor of in vivo clearance than is the industry standard approach of chemical synthesis followed by in vitro testing in a hepatocyte clearance assay. We believe that results like this study help support decisions at the FDA to move towards more AI-based computational models for advancing drug discovery and development.
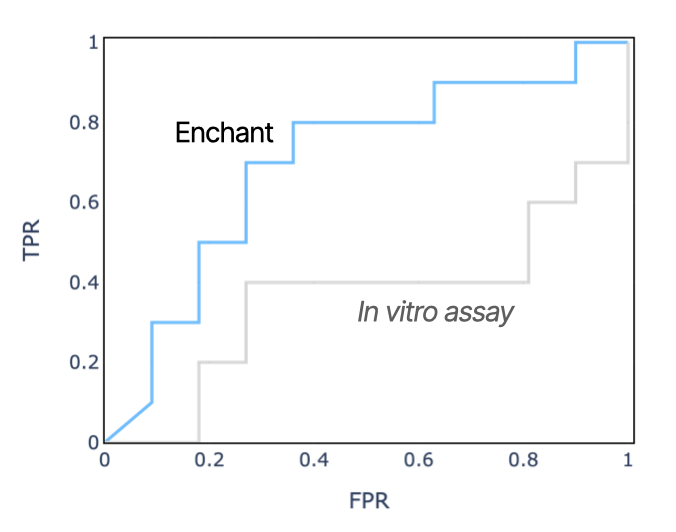
Scaling That Unlocks Future Potential
One of the most exciting aspects of Enchant is its scalability. By increasing model scale by over a factor of 10, Enchant v2 achieved clear and predictable gains in accuracy. Our scaling studies suggest that further improvements are well within reach, through model scale alone. In addition we continue to see improvements through investment in data engineering, model architecture, and improved fine-tuning frameworks.
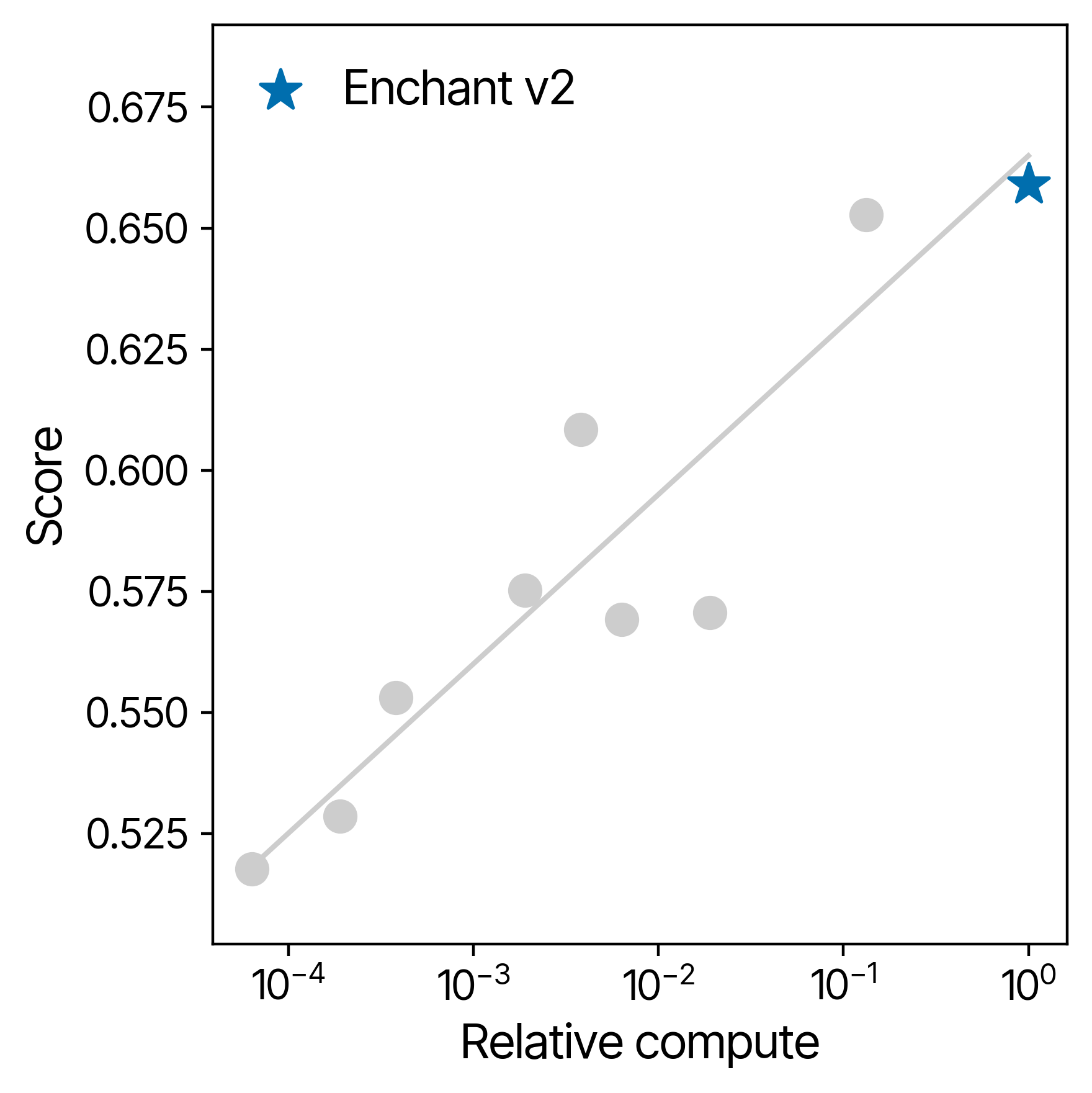
Like its predecessor, Enchant v2 is fine-tuned weekly on new experimental data across dozens of endpoints generated internally using Iambic’s automated chemical synthesis and experimentation platform. Enchant v1 completed training in early 2024. Enchant v2 training finished in Q1 2025. Enchant v1 was established as the leading predictive technology for drug discovery across a wide range of endpoints. Enchant v2 builds on that success, improves accuracy, and further widens the differentiation with respect to the field. We expect future releases of Enchant to continue to extend this frontier.
Conclusion
Enchant v2 marks a pivotal advance in the use of large-scale transformers for drug discovery. It validates a fundamental principle: with the right model, architecture, and training strategy, we can achieve exceptional predictive power even in the most data-constrained settings. By scaling systematically, we expect performance leaps to continue.
----------------------------------------------------------------------
1. All models were fine-tuned using identical train/test splits. The better of the two published TxGemma models was used, namely the 9B-parameter model. TxGemma was fine-tuned using Google DeepMind's published notebook.