Transforming Computational Drug Discovery with NeuralPLexer2

The binding between proteins and small molecule ligands is ubiquitous in life, and solving the three-dimensional structure of such protein-ligand complexes is crucial to both fundamental biological research and drug discovery. For instance, the modulation of cell signaling pathways – key drivers in many disease areas – entails delicate interactions among protein structures, and changes in their 3D shapes upon the addition of drug molecules. Determining these biomolecular structures and conformational changes on a computer has been a grand challenge for physical simulation tools and AI models like AlphaFold2.
In early 2023, we announced NeuralPLexer, a first-in-class generative AI model addressing this grand challenge problem of “jointly folding“ protein-ligand complex structures, and predicting their dynamics in a structure ensemble. The study, a collaboration between researchers from Iambic, Caltech and NVIDIA, has sparked great enthusiasm across the world [1, 2, 3, 4] for developing AI-based techniques for predicting and designing biomolecular structures. We are excited to announce that the methodology has been published today as an article in Nature Machine Intelligence.
At Iambic Therapeutics, immense progress has been made on NeuralPLexer in the year since its initial release.
A Leap in Model Capabilities
NeuralPLexer2, trained in October 2023, represents a significant improvement in the methodology. Apart from improving prediction accuracy for novel targets, we have also significantly expanded to scope of the model to include most categories of biological structures, adding protein-protein complexes, cofactors, post-translational modifications (PTMs), and protein-nucleic acid complexes, and encompassing almost all structures in the Protein Data Bank (PDB).
As the tip of the iceberg, NeuralPLexer2 shows excellent performance in predicting G protein-coupled receptors (GPCRs), a class of important therapeutic targets that challenge existing machine learning-based predictors. By solely taking their protein sequences and ligand chemical structures as inputs, on 32 recently determined GPCR structures, NeuralPLexer2 exhibits a median TM-score of 0.91 – an accuracy close to that of Nuclear Magnetic Resonance (NMR) experiments.
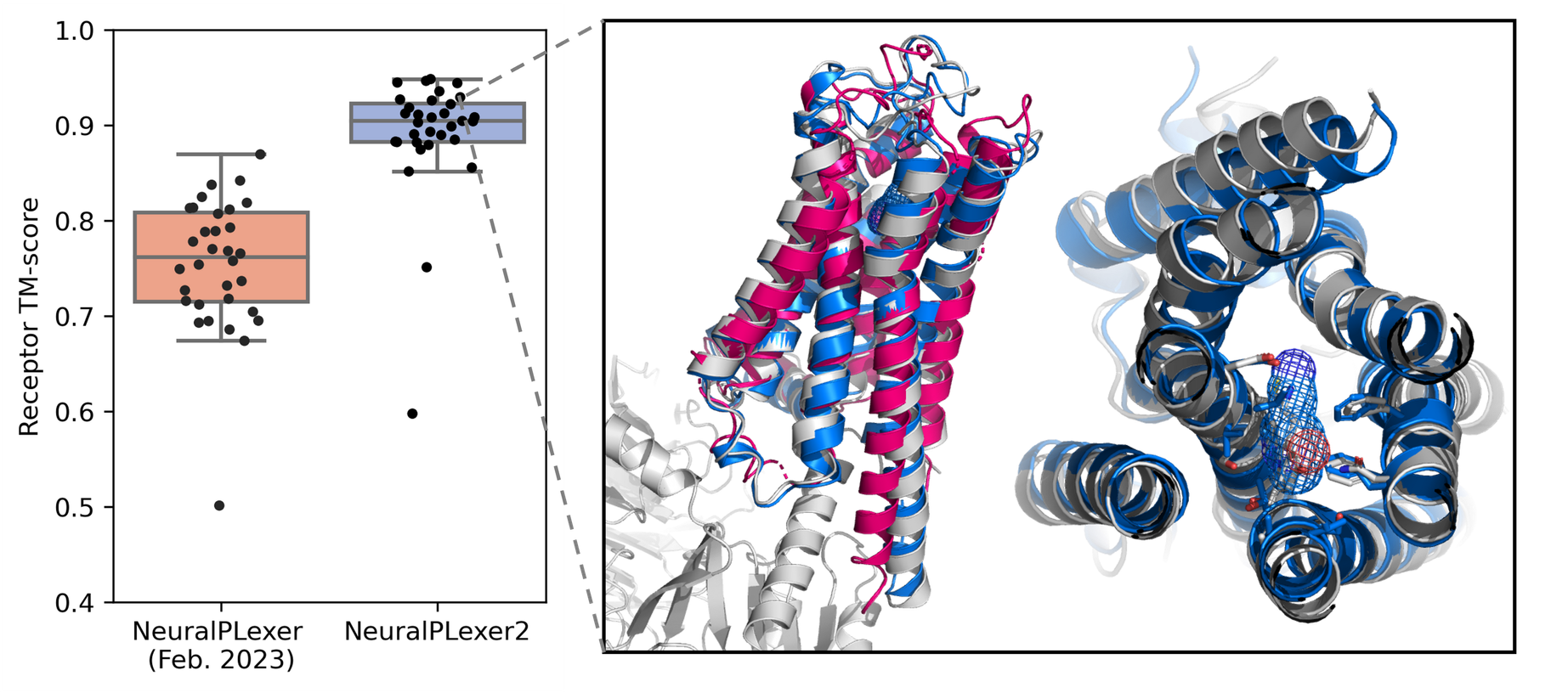
State-of-the-art Performance
NeuralPLexer2 shows superior performance on community-wide benchmarks for comparatively assessing the prediction of protein-ligand complex structures. On the PoseBusters benchmark for which all related PDB structures have been removed from the training data, NeuralPLexer stands out as the new standard for predicting protein-ligand binding:
- NeuralPlexer2 shows a substantially higher prediction success rate (defined by the percentage of ligand RMSD below 2 ångstrom versus experiment) compared to that of traditional molecular docking methods. Unlike traditional docking methods that require a reference ligand-bound (i.e. holo) protein structure, NeuralPLexer2 directly generates the 3D coordinates of the full binding complex end-to-end, and therefore can be readily applied to real-world applications where reference structures are typically unavailable.
- While full prediction can be performed based solely on protein sequence and ligand identity, performance can be further improved by specifying the site via a residue subset expected to be close to the ligand. Predictions on the PoseBusters set with site specified have a success rate of 76.8%.
- NeuralPLexer2 has built-in confidence estimation and incorporates physical constraints. For instance, on the subset of PoseBusters dataset where NeuralPLexer2 is confident in its predictions (175 out of 308 targets), NeuralPLexer2 achieves a success rate of 75.4% which is boosted to 93.2% when the candidate set of binding site residues (residues within 10Å of the ligand) is provided.
- High prediction speed. NeuralPLexer2 can be run on consumer-scale devices and with a significant inference throughput advantage relative to others. The roughly 50-fold acceleration relative to AlphaFold2 provides opportunities for very large scale in silico screening and proteome-wide studies of conformational response on ligand binding.

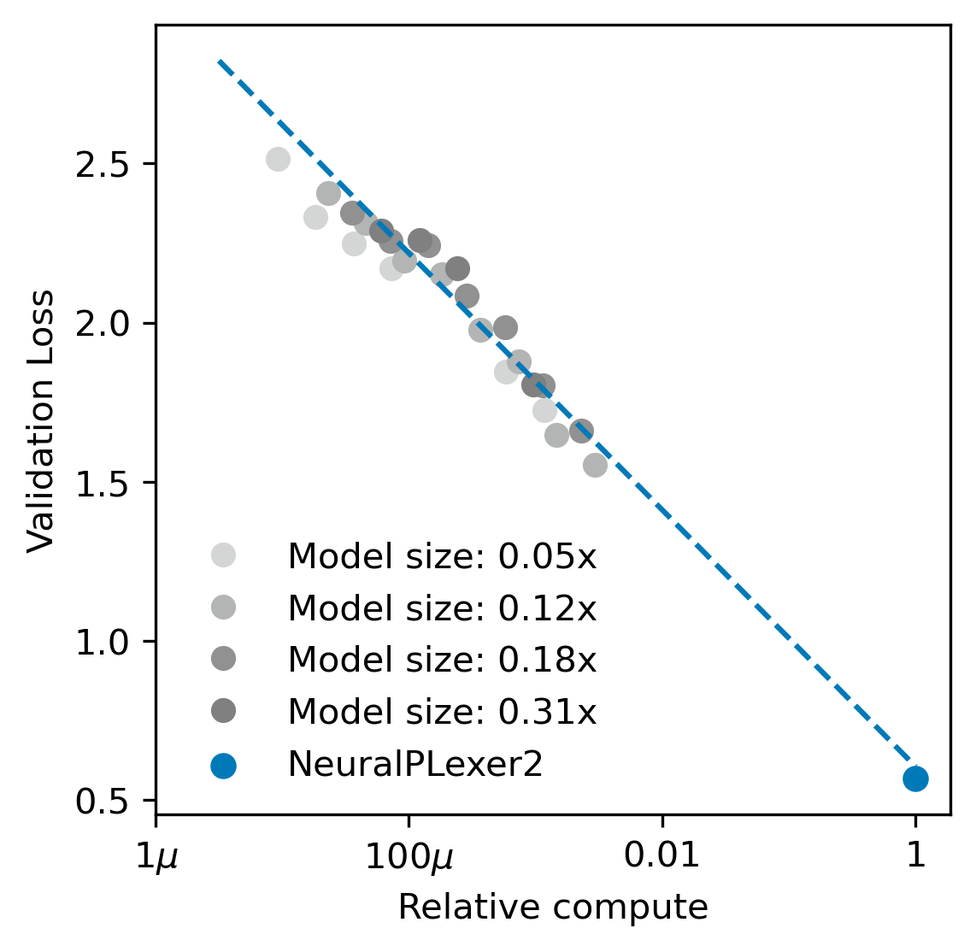
The technical pathway to NeuralPLexer2 and beyond
Underpinning NeuralPLexer2 is a tightly integrated team effort to overhaul the model architecture, data, and training pipelines. Below, we highlight a few technical accomplishments in NeuralPLexer2:
- Optimization. Hardware and memory-optimized geometrical attention building blocks were developed to support directly training the model on biological complexes with up to 2400 residues, and to enable higher inference throughput.
- Training data. The training data were expanded to fully encompass protein-protein complexes, protein-nucleic acid complexes, and to cover most post-translational modifications and cofactors. A data processing pipeline was carefully established to remove artifact ligands based on the atomic context. In summary, 134k refined structures from the PDB are used for training NeuralPLexer2, nearly doubling the training data compared to the original NeuralPLexer model.
- Model scaling laws. As the model’s generative head and the number of training iterations increase, we observed a predictable decay in the validation loss as shown by a linear fit in the log scale. Meanwhile, the generative modeling loss shows a good correlation with the binding site prediction accuracy calculated on predicted structures. Extrapolating the fitted scaling curves with respect to the total amount of compute leads to the final NeuralPLexer2 model, and we expect that further scaling along model size and data dimensions will continue to deliver improved performance.
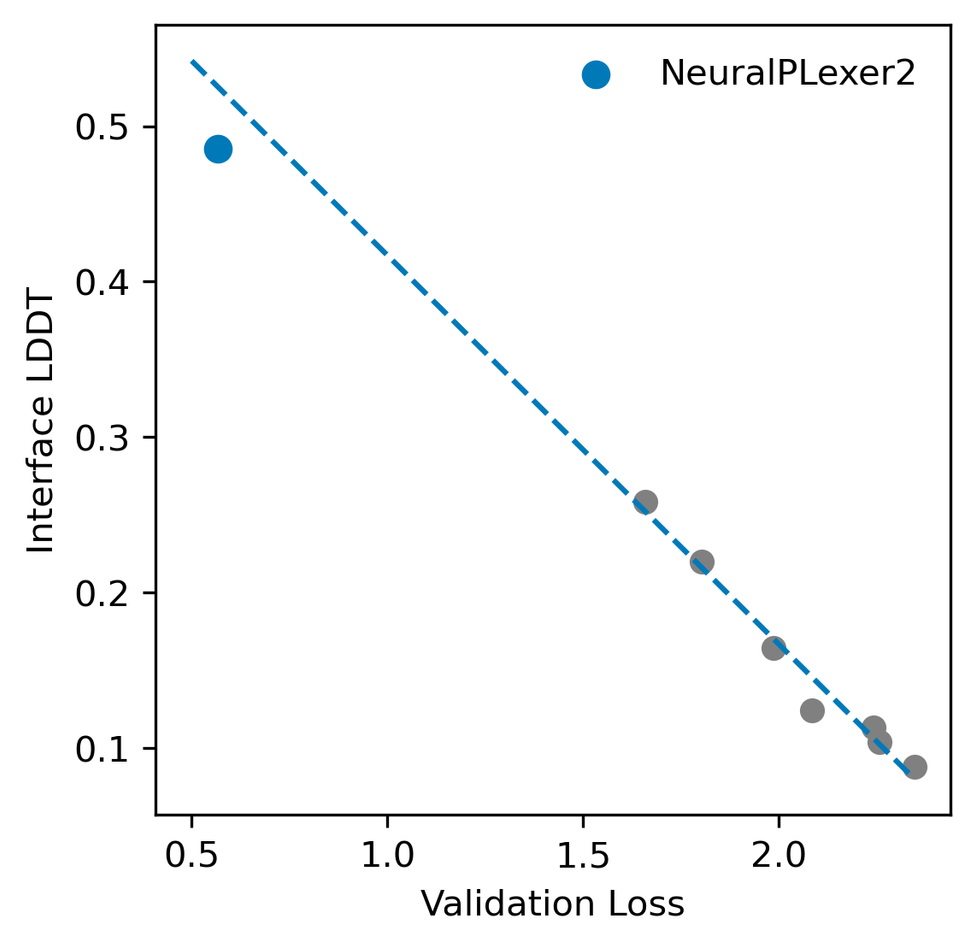
Leading the next wave of AI-driven drug discovery
At Iambic, NeuralPLexer has already shown the potential to unlock challenging targets, identifying cryptic sites, and elucidating complex mechanisms of action. By integrating NeuralPLexer2 into our technology platform, we have discovered pharmacological patterns for challenging targets that would have cost months to obtain via traditional experimental approaches. NeuralPLexer2 accelerates the process of compound design in our internal drug discovery programs, providing a hitherto inaccessible level of structural enablement via reliable prediction of protein-ligand complex structures for all compounds in a program. NeuralPLexer2 is our flagship technology for computational modeling of molecular biology, and we are confident in its capacity to transform structure-based drug discovery.